Rise of the Machine: When and How to Leverage Artificial Intelligence
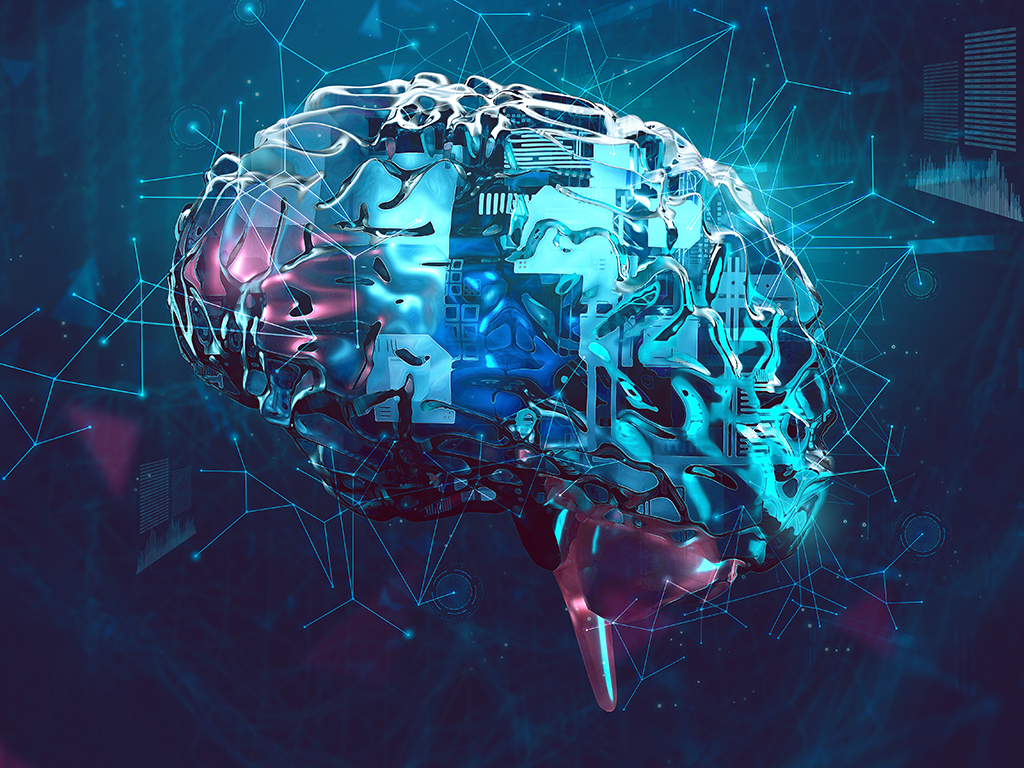
For decades, Artificial Intelligence (AI) lived only in the imaginations of novelists and filmmakers. For many, their first exposure to AI was in the film 2001, where an intelligent supercomputer (spoiler alert) murders human beings. Terminator: Rise of the Machines, along with numerous other films about killer droids and robots, didn’t assuage any fears about Artificial Intelligence.
Despite cinema’s warnings of AI gone awry, AI is here to stay, and it drives much of the technology used in our everyday lives. Here, Abe Kinney, director of product management at Alarm.com, and Charlie Erickson, chief technology officer of Stanley Security, define AI and discuss the challenges and opportunities these technologies bring to the security market.
Defining Machine Learning, Deep Learning, and AI
Arthur Samuel, a pioneer in computer science, defined AI in 1959 as a “field of study that gives computers the ability to learn without being explicitly programmed.” The two keys to AI are autonomy and adaptability, meaning an AI-enabled device can operate without continual human input and that it can adapt its behavior based on data it has collected itself.
Machine learning, which is a subcategory of AI, relies mainly on structured data – highly organized data that exists in a database – to make predictions. Though machine learning research was initially based on a knowledge-driven approach, in the 1990s there was a shift to a data-driven approach: scientists began building computer programs that analyzed large amounts of data and learned from the results. This led to deep learning becoming feasible in the 2010s.
Deep learning is a subcategory of machine learning that adds additional parameters and layers of processing to systems. It relies even less on human input, can use unstructured data, and can determine which features of the data are most important. We have deep learning to thank for credit card fraud detection, digital assistants, and self-driving cars.
Why Has Machine Learning Taken Off?
Several factors have contributed to the rising success of machine learning. First, data collection is increasing. “In the next three years there will be as much data collected as there were in the past thirty years,” Erickson says. “There is a wealth of data to train algorithms on. A lot of this data is coming from IoT devices, which we’re all installing.”
Secondly, computing power has increased dramatically. Graphics Processing Units (GPUs) have long been used in the security industry in H.264 and H.265 technology. “It turns out that they are great at doing intense parallel computing for machine learning,” Erickson continues.
Finally, in the past few years, developers have democratized the ability to generate machine learning algorithms. This results in an abundance of open-source algorithms – developers don’t need to build an entire algorithm from scratch to develop new solution. Erickson cites the Open Security Safety Alliance (OSSA) used by Bosch and the Axis Camera Application Platform as examples of open-source algorithms.
AI will continue to grow: private investors, the federal government, and consumers are seeing its worth. Private investment in AI was $70 billion in 2019 and the US government spent $6 billion in unclassified AI research in 2020. Recent polling shows that 40% of consumers believe that artificial intelligence improves their lives in some way.
AI Challenges
“AI is making hard things easy, but there are still some easy things that are hard,” Kinney quips. Machine learning applications are only as smart as the data that underpins them.
“Depending on what information is going in, you can build biases, you can have insights or patterns that you didn’t expect come to light in your model… Be mindful of how the technology is built and what it was trained on,” Kinney advises. “In the security industry, for example, using images from high mount cameras to identify people is different from training a machine learning algorithm from publicly-available Facebook photos or cellphone photos that were taken at eye level.”
Along with challenges come concerns. The charts here show what the worries and frustrations consumers have about AI. “It’s incumbent on those who develop the technology to truly have the technology provide a better experience,” Kinney said.
To instill trust in AI and do our part to protect privacy with AI applications, security installers should be “up front and transparent,” Kinney says. “Understand how to be compliant with biometrics and face recognition. Get customer consent to use data and to let their devices become smarter over time.”
Erickson points out that people creating AI technology have a high level of awareness of ethical issues, which has led to conversations in industry associations such as the IEEE about developing standards. It has also sparked legislation on data protection by governing bodies such as the EU.
AI Applications for Security
AI is changing the scope of security. Incident verification, for example, is becoming highly accurate through object classification, activity analysis, and alarm prioritization. Pixel-based motion detection, which had a long-standing reputation of false alarms, has given way to machine learning-enhanced motion detection that can examine what’s in the camera’s field of view and accurately classify it as an actual threat or just a nuisance. Security end-users are also discovering the benefit of using video surveillance to analyze business activity, through AI functionality such as people counting, heat mapping, crowd detection, and occupancy monitoring.
Additionally, “Audio is a rich data source we don’t pay enough attention to,” Erickson noted, but AI has brought audio to the forefront. This comes both in the form of classifying audio – whether it be the sound of a gunshot, breaking glass, or a person speaking under duress – and reducing alarms. AI-enabled devices can notice trends in day-to-day background noise and only send alerts when the audio is different from what it normally would be.
Avoiding the AI Bandwagon
When manufacturers claim to have AI enabled devices, sometimes it’s hard to tell if they are just jumping on the marketing bandwagon or if they truly have high-performing AI. To determine which is which, Erickson recommends that installers “talk to your vendor about how they’re testing the data sets they’re developing. Standards have not yet been established, so ask vendors which use cases and data sets they test against. Also look at the maturity of company that is developing the algorithm.”
Kinsey added that installers should do their own testing: “Test in your context, specific to your subset and customer types. Test real-world applications before you intend to deploy.”
As computing power continues to increase, AI use cases expand, and consumers become more comfortable, AI will carry on its trajectory from science fiction to reality.
Security professionals should educate themselves on the challenges of AI and its benefits in security applications to provide informed recommendations to their customers.
The “rise of the machine” has turned out to not be murderous droids but instead a world where the technologies that we depend on every day can perform more meaningfully for us. Now that’s a happy ending.